Projects
Smart, Connected, and Coordinated Maternal Care for Underserved Communities
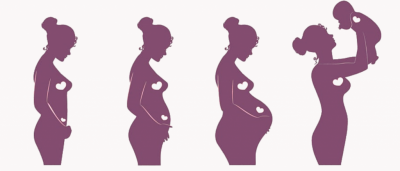
UNITE (UNderserved communITiEs) presents a community engagement model that is smart, deploying ubiquitous monitoring and lifelogging; connected, bringing together a diverse cast of community members including mothers, families, care providers, and outreach resources; and coordinated, using technology to proactively reach out to the community and use personalized intervention and education for improved self-management by the women. The UNITE project champions a model that is scalable in size, portable across different ethnic communities, and promises improved outcomes through better self-management and community enhanced motivational factors. The UNITE project performs a controlled study using a community of underserved Orange County mothers together with non-profit agencies, hospitals, and local support organizations to evaluate the efficacy of this new community-enhanced self-management approach, and its impact on community building. The project builds larger communities of healthcare providers, insurance providers, and governmental agencies that can work in concert to enhance the well-being and lifestyles of mothers and families across a diverse spectrum of socio-economically disadvantaged groups. The UNITE project also trains the next generation of healthcare providers to deploy socio-economically relevant Internet-of-Things (IoT) technology in a cost-effective and user-friendly manner.
Publications:
– Iman Azimi, Olugbenga Oti, Sina Labbaf, Hannakaisa Niela-Vilen, Anna Axelin, Nikil Dutt, Pasi Liljeberg, and Amir M. Rahmani, “Personalized Maternal Sleep Quality Assessment: An Objective IoT-based Longitudinal Study”, IEEE Access, 2019
– Holly Borg, Hannah Vasquez, Michelle Heredia, Melissa Navarrete, Nikil Dutt, Amir M. Rahmani, and Yuqing Guo, “A Self-Management Model: Using Wearable Devices for Continuous Monitoring during the Perinatal Period”, National Perinatal Association Annual Conference Perinatal Care and the 4th Trimester” (NPA’20), 2020
– Lucretia Williams, Gillian R. Hayes, Yuqing Guo, Amir M. Rahmani, Nikil Dutt, “HCI and mHealth Wearable Tech: A Multidisciplinary Research Challenge”, ACM CHI Conference on Human Factors in Computing Systems, Case Study (CHI’20-Case Study), 2020
_ Juho Laitala, Mingzhe Jiang, Elise Syrjälä, Emad Kasaeyan Naeini, Antti Airola, Amir M. Rahmani, Nikil D. Dutt, Pasi Liljeberg, “Robust ECG R-peak Detection Using LSTM”, The 35th ACM/SIGAPP Symposium On Applied Computing (SAC’20), 2019
Please refer to the UNITE website for more details: https://unite.ics.uci.edu
Mental Health Navigator
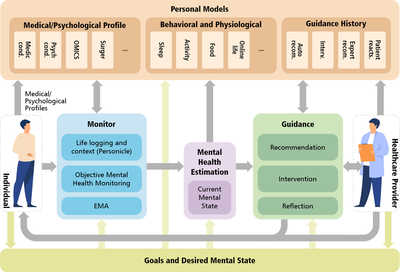
Traditionally, the regime of mental healthcare has followed an episodic psychotherapy model wherein patients seek care from a provider through a prescribed treatment plan developed over multiple provider visits. Recent advances in wearable and mobile technology have generated increased interest in digital mental healthcare that enables individuals to address episodic mental health symptoms. In the Mental Health Navigator project we are seeking to develop a comprehensive platform to measure, estimate, guide the individual’s mental health state within context of a therapist-in-the-loop, cybernetic goal-based system.
Articles:
Amir M. Rahmani, Jocelyn Lai, Salar Jafarlou, Asal Yunusova, Alex. P. Rivera, Sina Labbaf, Sirui Hu, Arman Anzanpour, Nikil Dutt, Ramesh Jain, Jessica L. Borelli, “Personal Mental Health Navigator: Harnessing the Power of Data, Personal Models, and Health Cybernetics to Promote Psychological Well-being”, arXiv, 2020
Jocelyn Lai, Amir Rahmani, Asal Yunusova, Alex Rivera, Sina Labbaf, Sirui Hu, Nikil Dutt, Ramesh Jain, Jessica Borelli, “Using Multi-modal Assessments to Capture Personalized Contexts of College Student Well-being in 2020: A Case Study”, JMIR Formative Research, 2021
Asal Yunusova, Jocelyn Lai, Alexander P. Rivera, Sirui Hu, Sina Labbaf, Amir M. Rahmani, Nikil D. Dutt, Ramesh C. Jain, & Jessica L. Borelli, “Assessing Mental Health in Emerging Adulthood through a Mental Health Navigator: A Study Protocol”, JMIR Journal of Research Protocols, 2021
COVID-19 Long-haulers
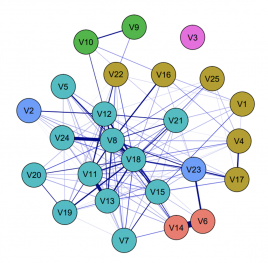
Emerging data suggest that the effects of infection with SARS-CoV-2 are far-reaching, extending beyond those with severe acute disease. Specifically, the presence of persistent symptoms after apparent resolution from COVID-19 has frequently been reported throughout the pandemic by individuals labeled as long-haulers. Long-haulers represent a very significant public health concern, and there are no guidelines to address their diagnosis and management. The objective of this project is to understand the long-term physical and mental influences of COVID-19 via data science tools. In this project, Using data collected from various sources, including electronic health records, social media posts, we study the differences in COVID-19 manifestation, the associations between long-haul symptoms, and risk factors that may contribute to becoming a COVID-19 long hauler.
ZotCare
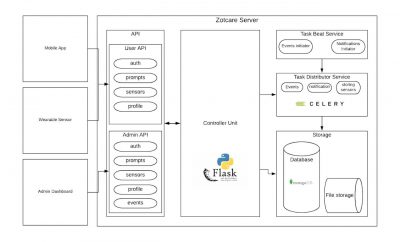
ZotCare is a dynamic and flexible multi-layer (sensor-smartphone-cloud) platform built as part of our NSF SCC UNITE project which provides a variety of services necessary to run a human study trial in one place. It is designed to support the entire life-cycle of a remote monitoring-intervention study from planning to deployment and analysis. It offers i) services to interface with wearable and portable sensors (both native and third party), ii) a multi-platform mobile app for two-way communication with users, collecting EMAs and self-reports, sending recommendations and reminders, and implementing remote interventions, iii) back-end services for storage, computation, control, and visualization, and iv) a web-based dashboard for workers, researchers, and end-users interact with the system.
A home-based, culturally and language specific intervention for dementia family caregivers: stress reduction and education with wearable technology for health
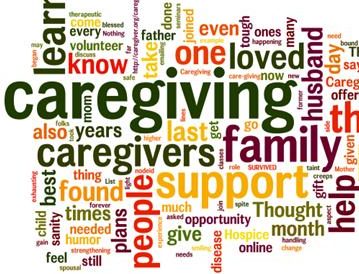
The $3.7M NIH R01 project entitled “A home-based, culturally and language specific intervention for dementia family caregivers: stress reduction and education with wearable technology for health” received funding from the National Institute of Aging (NIA). Jung-Ah Lee and Amir Rahmani are the PIs, and Lisa Gibbs, Adey Nyamathy, and Sanghyuk Shin are Co-Is of this project. The program aims to build caregiving and stress-management skills in family caregivers of persons with dementia or mild cognitive impairment. The study involves remote monitoring using wearable sensors, home visits, and follow-ups. This research is conducted in collaboration with Dr. Jung-Ah Lee’s group at UCI (https://faculty.sites.uci.edu/caregiverstudy/).
Publications:
– Jung-Ah Lee, Anthony Park, and Amir M. Rahmani, “Sleep Duration and Quality in Dementia Caregivers: Wearable Iot Technology,” Western Institute of Nursing’s 52nd Annual Communicating Nursing Research Conference (WINURSING’20), 2020, USA.
– Jung-Ah Lee, Sina Labbaf, Amir M. Rahmani, Priscilla Kehoe, and Nikil Dutt, “Wearable Internet-of-Things Technology: An Immigrant Dementia Caregivers Pilot Intervention,” The Alzheimer’s Association International Conference (AAIC’19), 2019, USA.
– Jung-Ah Lee, Kajung Hong, Jessica Borelli, and Amir M. Rahmani, “A Culturally Sensitive Dementia Family Caregiver Pilot Study,” Western Institute of Nursing’s 52nd Annual Communicating Nursing Research Conference (WINURSING’19), 2019, USA.
Research Partners:
Alzheimer’s Association Orange County Chapter,
Alzheimer’s Orange County,
Family Caregiver Resource Center,
Council of Aging,
Korean Community Services Health Center,
Southland Integrated Services, Inc
FoodLogging Platform
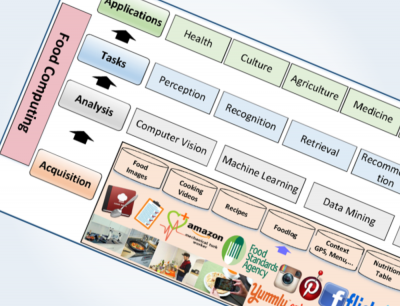
Models are built using data. Most successful search, social media, and recommendation systems are built using personal models to provide people the right information, at the right time, in the right context, usually even before a user articulates his need. Food recommendation systems need to be built using the same approach. A personal food model is essential for recommending the right food item at the right time. It is also essential to predict the effect of food so the right suggestions can be made to avoid unpleasant situations. We need to build such models using food logs collected for the person. Many applications for foodlogging are being developed based on detecting the dish or item being consumed and finding nutritional elements based on ingredients in these items. Detecting items and the volumes consumed requires a multimodal platform and nutritional data sources for items prepared using specific ingredients and recipes. To see more information please watch this YouTube demo.
Digital Health for Future of Community-Centered Care (D-CCC)
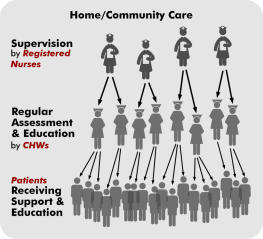
This project funded by the US National Science Foundation (NSF) focuses on the future of work in the context of community-centered care. The adhoc nature of the healthcare system in the USA necessitates an organized and affordable platform to increase the reach and services to the majority of the population. Currently, caregivers provide critical medical assistance in a community setting, particularly for elderly, disabled and vulnerable populations. Several states including California employ a community healthcare worker (CHW) initiative as an alternative to registered health care professionals to extend the reach of home-delivered services. While there is a critical need to scale and personalize these services, it faces significant challenges in the face of the increasing number of elderly, disabled and vulnerable communities requiring medical assistance, coupled with low wages for caregivers and rapidly increasing demands for skilled labor. The existing community-centered care (CCC) model is led by registered nurses (RNs) who train and supervise the CHWs, who in turn provide the necessary care for the community. This CCC model suffers from an inability to scale in a cost-effective manner while providing personalized and quality care for the community. The project proposes the digital health enabled community-centered care (D-CCC) model to transform the manual, restricted, and unstructured state of the current community healthcare landscape into a scalable, digital, and automated space. See more information here.
Research Team:
* Amir Rahmani
* Adey Nyamathi
* Nikil Dutt
* Pramod Khargonekar
* Pamela Austin
* Anil Kanduri
* Sara Rodrigues
Holistic Stress Reduction in Adolescents through Multi-modal Personal Chronicles
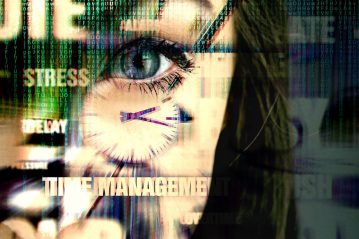
Emotional stress is a major factor contributing to the leading causes of death in the United States. Adolescence is a particularly risky period of development during which rates of mental disorders and mortality increase dramatically, changes which may in part be due to rapid shifts in physical and psychological development in the context of brain immaturing. Stress management and reduction are crucial capacities for adolescents since they often experience high-intensity negative emotions and lack the maturity required to exert top-down control over these intense emotional experiences. Effective stress management techniques for adolescents must meet them where they live — on their smartphones– and engage them as active participants in their health assessment/promotion. This proposal aims to develop a holistic stress reduction strategy combining emerging IoT technologies with a multi-modal lifelogging framework that enables psychologists and caregivers to identify the root causes of stress and build an evidence-based approach to monitoring stress and emotion in adolescents.
Intelligent and Automatic Pain Assessment Tool Employing Behavioral and Physiologic Indicators
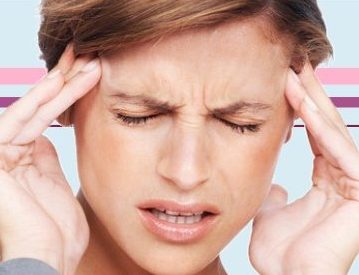
Pain is an unpleasant sensory and emotional experience associated with actual or potential tissue damage or described in terms of such damage. It is a subjective sensation and patients self-report is considered the most reliable indicator of pain. However, assessment of pain is particularly difficult when the ability of the patient to communicate is limited or impossible e.g. during critical illness, under sedation and anesthesia or for infants.
The objective of this project is to benefit from the offered features of the IoT and sensor networks to provide an automatic tool which can detect and assess pain employing behavioral and physiologic indicators such as facial muscle activity, heart rate, blood pressure, and breathing rate. The aim of this project is to develop a system based on Internet of Things to detect and assess pain in a reliable and objective way by enabling the pain diagnoses in the case when the patient is unable to communicate and express the pain sensations.
Research Partners:
School of Nursing, University of California, Irvine, USA
UCI Medical Center, University of California, Irvine, USA
Department of Computer Science, University of California, Irvine, USA
Department of Informatics, University of California, Irvine, USA
Department of Future Technologies, University of Turku, Finland
Department of Nursing Science, University of Turku, Finland
Department of Anesthesiology and Intensive Care Medicine, University of Turku, Finland
Division of Perioperative Services, Intensive Care Medicine and Pain Management, Turku University Hospital, Finland
Center for Functional Materials (FUNMAT), Åbo Akademi University, Finland
Internet of Cognitive Things for Personalized Healthcare
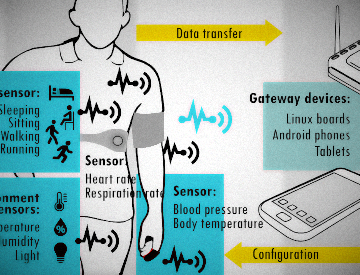
Quality of Experience (QoE) is a key metric for the successful delivery of end-user services for IoT-enabled applications. Achieving consistent end-user QoE poses tremendous challenges in the face of resource constraints and dynamic variations at multiple scales of the IoT system stack: at the application, network, resource, and device levels. This proposal outlines a self-aware cognitive architecture – the Internet of Cognitive Things (IoCT) – that delivers acceptable QoE by adapting to dynamic variations in infrastructural compute, communication and resource needs, while also synergistically learning and adapting to end user behavior. The approach leverages edge (i.e., Fog) computing architectures to introduce intelligence and adaptability in integrated multi-scale IoT systems. The objective is to efficiently manage information acquisition, communication and processing across different scales of the IoT systems, while synergistically coupling learning of end-user behaviors to deliver efficient and customized services. The proposed IoCT system is the first example of architecture where a network of algorithms communicates and collaborates synergistically to achieve a system-wide objective. Cognition and edge computing architectures are leveraged to introduce intelligence and adaptability in integrated multi-scale IoT systems, through a Personal Holistic Cognitive Optimization (PHCO) framework. To this aim, the IoCT will adopt recently proposed learning and control techniques (i.e. Deep Q-Networks), and exploit self-awareness principles to achieve effective system optimization. The project leverages on-going collaboration with the Turku University Hospital to demonstrate a personalized ubiquitous healthcare framework using the Early Warning Score (EWS) system for human health monitoring. Healthcare spending accounts for almost 17% of the GDP in the US. In healthcare, effective monitoring and observation of patients plays a key role in detecting a deteriorating patient. This project’s exemplar application on efficient early detection of these life-threatening signs can potentially save lives through better quality of care, and timely delivery of critical/urgent health indicators. The framework and services are also applicable to a broad range of other IoT application domains.
A joint project between the Academy of Finland and National Science Foundation (NSF), US.
Link to Academy of Finland press release (available only in Finnish)
http://www.aka.fi/fi/akatemia/media/Tiedotteet1/2017/suomen-akatemia-ja-yhdysvaltojen-kansallinen-tiedesaatio-nsf-rahoittavat-yhdessa-langattoman-tietoliikenteen-tutkimushankkeita/